The Optimization Module for MembranePlus extends and enhances the program’s basic capabilities in several important ways. It uses a powerful direct search optimization algorithm to minimize an objective function (error function) that you specify within the MembranePlus framework. In simple terms, the optimizer adjusts your selected set of model parameters in order to minimize the difference between predicted and observed values of the drug concentrations from your in vitro permeability or hepatocytes studies.
Using your measured concentration vs. time data, fit parameters to build more robust models (e.g., nonlinear kinetics) and “learn” from your chemical series. Or, apply the feature to help design your in vitro permeability (Caco-2, PAMPA, MDCK) or hepatocyte experiment – based on your compound’s properties, how long do you need to run the experiment? At what pH? Or what shaking rate?
-
Model Building - Unlock Key Information...
As a model building tool, the Optimization Module allows you to calibrate simulation models to best match your experimental data.
Building a general model from a set of membrane experiments is accomplished by first assembling a database for which experimental data are available. With this data for a significant number of membrane experiments, you can then select a set of parameters to be optimized (fitted) to make the simulation match the data as closely as possible. This allows you to then ‘unlock’ important information that may have been overlooked when the initial data sets were measured, and you can better inform your GastroPlus® PBPK model inputs to predict in vivo exposure with confidence.
-
Experimental Design - Utilize Your Resources Better...
As a design tool, the Optimization Module provides the ability to determine optimum values for various parameters that define a membrane or hepatocyte system and experiments in order to match a desired concentration-time profile or a set of concentration-time profiles. You can also leverage the Optimization Module to answer a series of ‘what if?’ questions – e.g., if my compound is a candidate for lysosomal trapping, how much do I need to adjust the intracellular pH in my experiment to capture the effect?
Model parameters can be fitted to data for a single record, or across multiple records simultaneously.
The program will run one simulation for each record each time it changes the value(s) of one or more model parameters. Typically, hundreds of iterations will be performed, each with N simulations, where N is the number of records whose observations are being used to compare predicted and observed values. Objective function weighting is user-defined, and includes the most common weighting schemes.
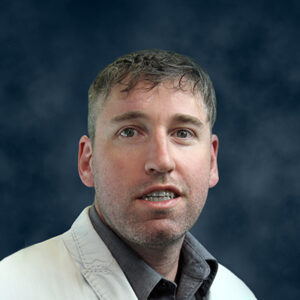
James Mullin
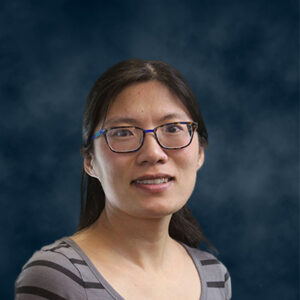
Haiying Zhou